- Cybermind Nexus
- Posts
- The Trillion Dollar Cloud War
The Trillion Dollar Cloud War
AI Supremacy Battle

Welcome to the Sunday Edition!
Hello,
Welcome to this special Sunday edition of "Cybermind Nexus." We're diving deep into a topic that has been brewing since our Wednesday announcement.
In the vast world of artificial intelligence, tech titans like Google, Microsoft, and Amazon are locked in a fierce battle for supremacy. Their focus areas are cloud computing and AI chips, with massive investments aimed at securing a pivotal role in hosting, processing, and optimizing AI models.
This battle has significant implications, shaping the future of AI applications for businesses, governments, and consumers alike.
Cloud providers, such as Amazon Web Services (AWS) and Microsoft, are teaming up with AI startups to capture revenue as AI adoption surges. In the realm of AI chips, Nvidia currently leads, but Google, Microsoft, and Amazon are in hot pursuit.
Enterprises seeking to adopt AI face various challenges, including model selection, cost optimization, integration, and talent gaps. Building robust internal AI capabilities is crucial for overcoming these hurdles.
As the AI landscape evolves, the next phase of competition will center on optimization, deployment, and adoption challenges. The winners will be those who can turn AI innovations into tangible business value.
Join us as we explore the intriguing dynamics of this transformative era in AI. Will the tech giants maintain their dominance, or will new stars rise? Find out in this special Sunday edition of Cybermind Nexus.
Tom
P.S. If you like what you're reading, why not forward this newsletter to a friend?
P.P.S. Did someone awesome forward you this newsletter? Sign up here.
AI SUPREMACY BATTLE
The Trillion Dollar Cloud War

The world of artificial intelligence is rapidly evolving, driven largely by fierce competition between major tech giants like Google, Microsoft, Amazon and more. These companies are locked in a high-stakes battle to develop the best AI capabilities and dominate the market.
At the center of this battle are two key components that form the infrastructure to develop, train and deploy advanced AI models: cloud computing and computer chips. By investing heavily in these areas, tech companies aim to gain a competitive edge to host, process and optimize the next generation of artificial intelligence.
The result is a fascinating AI arms race, with each company racing to build the largest and most powerful AI supercomputers, hire the top AI talent, and partner with the leading AI startups. Huge amounts are being spent to nurture proprietary AI chips and expand cloud server farms running complex neural networks.
This battle for AI supremacy has massive implications, as AI is poised to transform major industries and sectors. The companies that succeed in this race will be at the forefront of shaping how AI evolves and is applied for business, government and consumers. They may gain unmatched insights from data that fuel innovation and new products, or capabilities that disrupt their competitors.
In this high-stakes environment, every move matters. Acquisitions of AI startups, development of custom silicon chips, expansion of cloud infrastructure - these are all strategic plays in the unfolding quest to win at AI. This battle will likely intensify as more real-world AI applications emerge.
The question is, which companies will come out on top?
SPONSORED BY: IT COULD BE YOU
Amplify Your Impact with Cybermind Nexus Sponsorship
Are you looking to connect with an audience passionate about the psychology of AI and cybersecurity? Partnering as a sponsor with Cybermind Nexus places your brand at the heart of these dynamic, cutting-edge discussions.
Cloud Wars

The major cloud providers are using strategic investments and partnerships in AI to drive revenue growth for their cloud platforms. As companies like Anthropic, Cohere, and others build large language models and make them commercially available, cloud usage goes up significantly.
For example, Anthropic has committed to using AWS as its primary cloud provider. As Anthropic's large language model Claude gains more enterprise adoption, this could lead to billions in extra revenue for Amazon Web Services. By investing in Anthropic early, Amazon has positioned itself to benefit financially as Claude scales up.
Microsoft is taking a similar approach with its investment in OpenAI, the maker of ChatGPT. By integrating OpenAI models like GPT-3 into Azure, Microsoft strengthens its AI offerings and makes Azure more appealing to customers who want to leverage large language models. As ChatGPT and future OpenAI products gain popularity, Microsoft is set up to reap higher Azure usage and revenue.
This pattern is playing out across the major cloud platforms. Google Cloud has invested heavily in Anthropic as well, up to $2 billion. And partnerships with AI startups allow cloud providers to capture value as new models drive up demand for compute power and cloud services.
The cloud wars are intensifying as providers hedge their bets across promising AI startups and models. But it also creates a cycle where AI innovation helps public cloud revenue grow. As these language models continue to improve and gain adoption, they will funnel significant business back to the platforms they are built on top of.
Chip Wars
The development of specialized AI chips is another key battleground among tech giants. AI models require immense computing power, which has spurred companies to design their own custom silicon for optimal performance.
Nvidia has dominated the AI chip market with its GPUs to date. However, competitors are rapidly developing new offerings to challenge Nvidia's position:
Nvidia - Creator of the GPU and leader in AI chips. Recently announced the H100 chip and upcoming H200 chip specifically optimized for AI workloads.
Microsoft - Unveiled its homegrown Maia 100 chip in November 2022, designed to run large AI models efficiently. Expected to be available in early 2024.
Amazon Web Services - Announced the new Trainium 2 chip in November 2022, the next generation of its AWS-designed chips for machine learning.
Google - Developing its Tensor Processing Unit (TPU) chips in-house for years to power its own services. Recently unveiled the TPU v5 chips focused on AI tasks.
Chip innovation is ramping up to meet the demands of increasingly large AI models. While Nvidia still commands the market, major players are investing heavily in custom silicon to potentially challenge its leadership position. The next 2-3 years will see rapid advancement and competition in the AI chip space.
The AI Chip Landscape
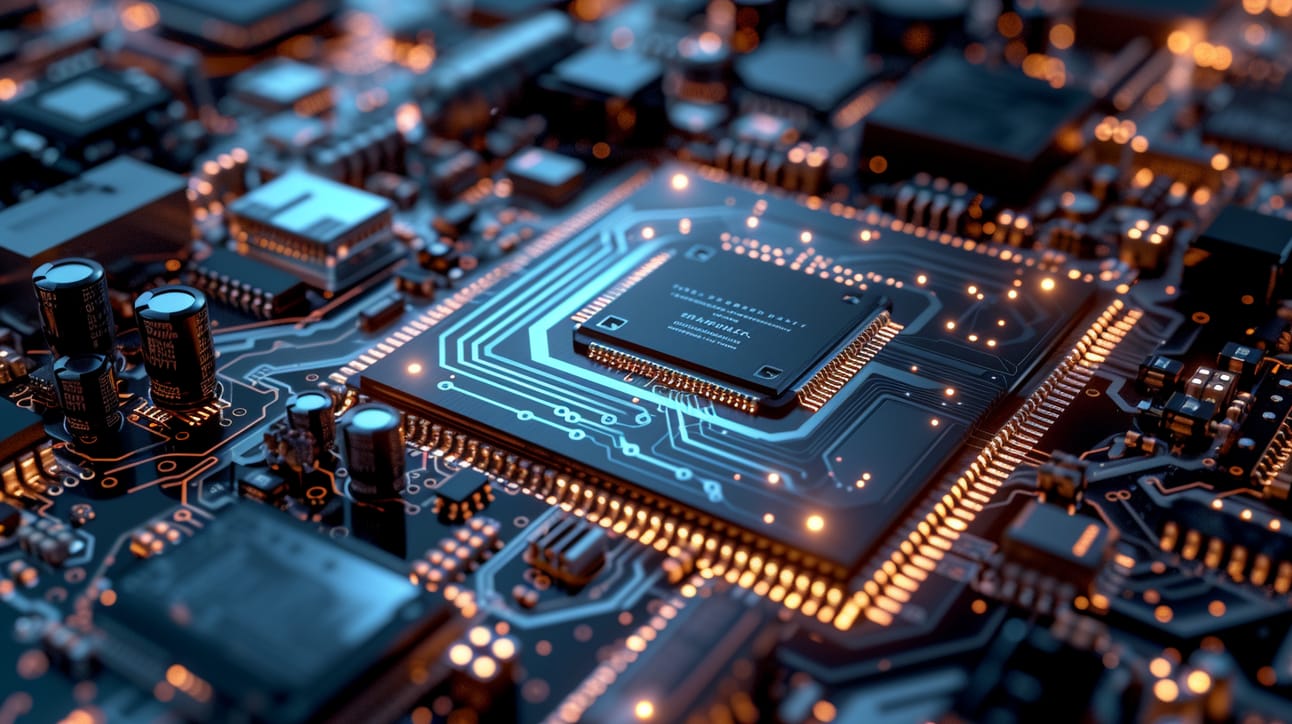
The AI chip landscape is rapidly evolving as technology giants and startups compete to develop specialized hardware for training and deploying large language models.
Currently, Nvidia dominates the market with its GPUs optimized for AI workloads. The A100 GPU introduced in 2020 delivers unmatched performance for training large models. Meanwhile, Nvidia's Turbocharged Inference Engine on its T4 Tensor Core GPUs accelerates deployment of pretrained AI models.
However, competitors like Google, Microsoft, Amazon and startups like SambaNova and Cerebras Systems aim to challenge Nvidia's dominance.
Google'sTensor Processing Units (TPUs) are tailored for its models and services, providing significant speed and efficiency gains. The 5th generation TPUv5 chips promise nearly double the performance of the prior generation.
Microsoft recently announced its Maia 100 chip designed for large language model training and inferencing. The Maia 100 leverages a novel architecture to optimize matrix multiplication operations critical for AI workloads.
AWS Trainium and Graviton chips equip its cloud with high performance custom silicon for AI. The recently announced Trainium 2 promises significant leaps in training performance.
Startups like SambaNova take a software-first approach using advances like model parallelism and novel dataflow architecture to extract maximum efficiency from AI chips. Cerebras's enormous Wafer Scale Engine packs 850,000 AI cores on a single chip enabling extreme parallelism.
As the AI chip space rapidly evolves, there is ample room for innovation in novel architectures, advanced packaging, and software to enable faster, more powerful and efficient AI. The next few years will likely see heated competition and many new breakthroughs in this critical hardware domain underpinning the AI revolution.
AI Chip Innovation Timeline
The AI chip landscape is rapidly evolving with major tech companies making key announcements about new hardware designed specifically for AI workloads. Here's a look at some of the recent timeline of AI chip innovation from the major players:
2010-2011: During this period, GPUs (Graphics Processing Units) began to be widely recognized for their effectiveness in accelerating AI tasks, especially deep learning algorithms.
2012: NVIDIA's Kepler GPU architecture was released. Kepler GPUs were significant for AI due to their high computational power and efficiency, which made them suitable for deep learning tasks.
2014: NVIDIA launched the Tegra K1 SoC (System on Chip), which integrated a Kepler-based GPU. This was an important development for bringing powerful AI computation to mobile and embedded devices.
2016: Google announced the Tensor Processing Unit (TPU), a custom AI accelerator chip designed specifically for neural network machine learning.
2017: NVIDIA introduced Volta GPU architecture, which included Tensor Cores optimized for deep learning. These Tensor Cores accelerated mixed-precision matrix multiplication operations, a common operation in neural network training and inference.
2018: Graphcore, a UK-based company, released its Intelligence Processing Unit (IPU), a new type of processor specifically designed for machine intelligence.
2019: Intel launched Nervana NNP-I, an AI chip designed for inference tasks. They also acquired Habana Labs, a company specializing in AI processors, to boost their AI chip development.
2020: Apple announced its M1 chip, which included a dedicated Neural Engine for AI and machine learning tasks.
2021: Google introduced the third generation of its Tensor Processing Units (TPU v4), which offered significant improvements in performance for machine learning workloads.
2022: NVIDIA released the Hopper GPU architecture, which further enhanced AI computing capabilities, especially in terms of AI model training and inference.
2023-2024: Continued advancements in AI chip technology, focusing on increasing efficiency, reducing power consumption, and enhancing the capability of edge AI applications.
Potential for Smaller Companies

Despite the tech giants seemingly dominating the AI landscape, there remain opportunities for smaller companies to make an impact. Here are some of the ways that startups and emerging players could gain a foothold:
Focusing on vertical or niche applications rather than general AI. Smaller teams can build expertise and deliver specialized AI solutions tailored to specific industries or use cases.
Leveraging open source models and frameworks like HuggingFace and PyTorch. Rather than building models from scratch, smaller companies can fine-tune and optimize existing architectures.
Providing services and support around AI deployment and integration. Helping enterprises implement and scale AI is in high demand.
Building developer-focused tools and platforms. Creating the tools that empower engineers and researchers to build with AI.
Exploring innovative business models like code generation as a service. New ways of monetizing AI capabilities.
Getting acquired by the tech giants seeking access to talent and technology. Large companies are aggressively acquiring AI startups.
Partnering with bigger players early on. Collaborating on research, datasets, and compute resources.
Focusing on transparency, ethics and responsible AI practices. This gives startups a brand differentiation.
Leveraging geographic concentrations of talent and support programs. Certain innovation hubs assist new ventures.
Crowdfunding and alternative sources of capital. Democratizing access to early stage funding.
While challenging, nimble startups have paths to carving out market share in AI. With creativity and focus, they can provide value amidst intense competition.
Optimization and Deployment Challenges
Enterprises looking to adopt AI face difficulties optimizing models and deploying solutions amidst the rapidly evolving landscape. As new chips, cloud offerings and models emerge, companies must navigate tradeoffs around performance, accuracy, cost and other factors.
Several key challenges stand out:
Model Selection: With new models frequently released from vendors like Anthropic, Cohere, Google, etc., enterprises must evaluate options and select the right model for their needs. This requires benchmarking on relevant datasets and workflows.
Cost Optimization: Running large models on cloud platforms can incur high costs. Companies need strategies to right-size models, share compute resources across teams, and select cost-effective deployment options.
Integration: Most enterprises have complex existing infrastructure. Integrating and deploying AI solutions within legacy systems can require extensive development work.
Explainability: For many applications, being able to explain model outputs is critical. Companies must implement techniques like LIME to ensure model explainability.
Monitoring: Once in production, models require ongoing monitoring, re-training and optimization. Tools and expertise are needed to collect production data and feedback loops.
Talent Gaps: All of the above requires rare skills in AI engineering, MLOps, chip optimization and data science. Acquiring and growing this talent remains extremely challenging.
While exciting new AI capabilities arrive daily, turning cutting-edge research into production business value remains difficult. Companies that build strong internal capabilities in AI engineering and MLOps can gain a competitive advantage by efficiently navigating the turbulent waters of AI innovation.
SPONSORED BY: IT COULD BE YOU
Amplify Your Impact with Cybermind Nexus Sponsorship
Are you looking to connect with an audience passionate about the psychology of AI and cybersecurity? Partnering as a sponsor with Cybermind Nexus places your brand at the heart of these dynamic, cutting-edge discussions.
Final Thought

The AI landscape is rapidly evolving, with tech giants making big bets to establish dominance in cloud services and chips optimized for AI workloads. This competition is heating up, as evidenced by the slew of recent chip announcements from Nvidia, Microsoft, Amazon and Google.
Nvidia has enjoyed market leadership, but challengers are emerging. In addition to the big tech companies, startups like SambaNova Systems and established players like Intel aim to take a piece of the AI chip pie. Much of this innovation is focused on increasing memory bandwidth and capacity to better support large language models and other AI applications.
It remains to be seen which companies will come out ahead in the cloud and chip wars. Amazon stands apart as the only major cloud provider without an AI chip announcement, instead relying on partnerships. However, AWS' scale and its new Graviton chips make it a formidable contender. Meanwhile, Microsoft and Google benefit from synergies between their cloud and AI divisions.
I predict the next phase of competition will center around optimization, deployment and adoption challenges. Companies that can translate AI innovations into tangible business value will have an edge. Consultancies and startups playing an integration role will thrive. There are also opportunities for smaller, disruptive companies, especially in open source AI development.
Exciting times lie ahead as these technologies continue maturing. The winners will be those who can operationalize AI to solve real-world problems.
How did you like this edition of Cybermind Nexus? |
